VCMaster: Generating Diverse and Fluent Live Video Comments Based on Multimodal Contexts
ACM MM 2023
Abstract
Live video commenting, or "bullet screen," is a popular social style on video platforms. Automatic live commenting has been explored as a promising approach to enhance the appeal of videos. However, existing methods neglect the diversity of generated sentences, limiting the potential to obtain human-like comments. In this paper, we introduce a novel framework called "VCMaster" for multimodal live video comments generation, which balances the diversity and quality of generated comments to create human-like sentences. We involve images, subtitles, and contextual comments as inputs to better understand complex video contexts. Then, we propose an effective Hierarchical Cross-Fusion Decoder to integrate high-quality trimodal feature representations by cross-fusing critical information from previous layers. Additionally, we develop a Sentence-Level Contrastive Loss to enlarge the distance between generated and contextual comments by contrastive learning. It helps the model to avoid the pitfall of simply imitating provided contextual comments and losing creativity, encouraging the model to achieve more diverse comments while maintaining high quality. We also construct a large-scale multimodal live video comments dataset with 292,507 comments and three sub-datasets that cover nine general categories. Extensive experiments demonstrate that our model achieves a level of human-like language expression and remarkably fluent, diverse, and engaging generated comments compared to baselines.
Method
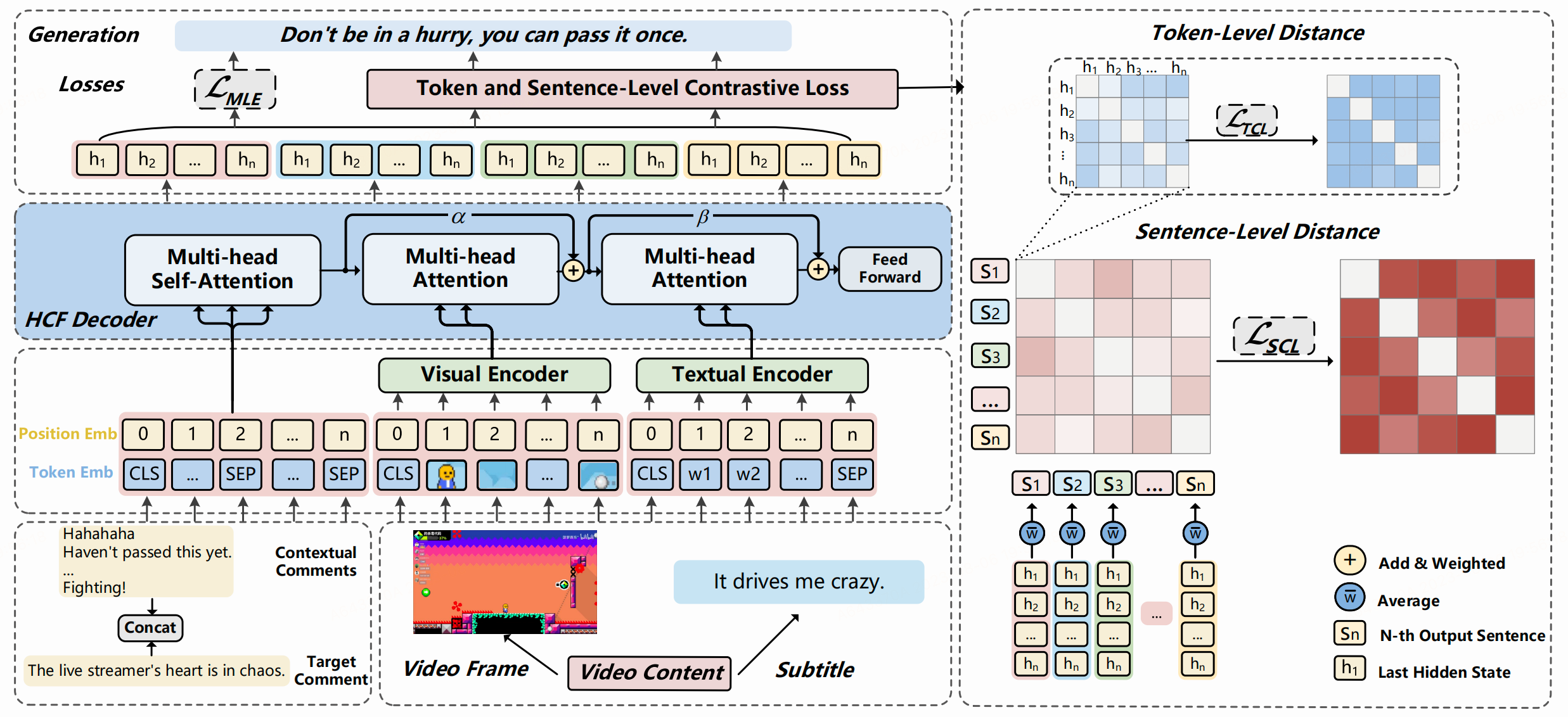
Overview of VCMaster, the proposed Multimodal Live Video Comments Generation model that generates fluent and diverse human-like comments based on video frames, subtitles, and contextual comments. \mathcal{L}_{TCL} and \mathcal{L}_{SCL} aim to enlarge the distance between tokens and sentences, improving the diversity of generated comments at both the token-level and sentence-level. Heatmaps indicate the degree of similarity, with darker colors representing greater distance and lower similarity.
The live video comment generation task aims to generate human-like comments by understanding human behaviour. Typically, people post their comments according to current video content or contextual comments from other viewers. In this study, We propose a novel approach named "VCMaster" for multimodal live video comments generation, which involves three modalities to enrich the video information in complex social scenarios and focuses on improving generation quality and diversity, as depicted in Figure 1.
We introduce a Hierarchical Cross-Fusion(HCF) Decoder to minimize the information loss during modality input and feature extraction process. Additionally, considering the consistency of the text structure of contextual comments with groundtruth comments, we decode contextual comments together with groundtruth in decoder and compute their attention weights by self-attention module. We also introduce a Sentence-Level Contrastive Loss (
BibTeX
@inproceedings{zhang2023vcmaster,
title={VCMaster: Generating Diverse and Fluent Live Video Comments Based on Multimodal Contexts},
author={Zhang, Manman and Luo, Ge and Ma, Yuchen and Li, Sheng and Qian, Zhenxing and Zhang, Xinpeng},
booktitle={Proceedings of the 31th ACM International Conference on Multimedia},
year={2023}
}